LETTER FROM THE EDITOR |
Dear Colleague,
Tomorrow, Thursday, November 10 at 9 am PT, Perceive will deliver the free webinar “Putting Activations on a Diet – Or Why Watching Your Weights Is Not Enough” in partnership with the Edge AI and Vision Alliance. To reduce the memory requirements of neural networks, researchers have proposed numerous heuristics for compressing weights. Lower precision, sparsity, weight sharing and various other schemes shrink the memory needed by the neural network’s weights or program. Unfortunately, during network execution, memory use is usually dominated by activations–the data flowing through the network–rather than weights.
Although lower precision can reduce activation memory somewhat, more extreme steps are required in order to enable large networks to run efficiently with small memory footprints. Fortunately, the underlying information content of activations is often modest, so novel compression strategies can dramatically widen the range of networks executable on constrained hardware. Steve Teig, Founder and CEO of Perceive, will introduce some new strategies for compressing activations, sharply reducing their memory footprint. A question-and-answer session will follow the presentation. For more information and to register, please see the event page.
For the past eight years, the Alliance has surveyed developers of vision-based products to gain insights into their choices of techniques, languages, algorithms, tools, processors and APIs, as well as understand product development challenges and trends. Please help us by taking a few minutes to share your thoughts in the 9th annual Computer Vision Developer Survey. In return you’ll get access to detailed results and a $50 discount on the Embedded Vision Summit in May 2023. You’ll also be entered into a raffle to win one of 20 Amazon Gift Cards worth $50! Click here to take the survey now.
Brian Dipert
Editor-In-Chief, Edge AI and Vision Alliance |
FLEXIBLE ARCHITECTURES FOR PROCESSING ACCELERATION |
Accelerate Tomorrow’s Models with Lattice FPGAs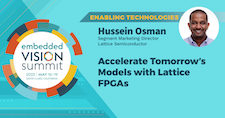
Deep learning models are advancing at a dizzying pace, creating difficult dilemmas for system developers. When you begin developing an edge AI system, you select the best available model for your needs. But by the time you’re ready to deploy your product, your original model is obsolete. You’d like to upgrade your model, but your neural network accelerator was designed with previous-generation models in mind and struggles to deliver top performance and efficiency on state-of-the-art models. The solution is hardware that adapts to the needs of whatever algorithms you choose. Hardware programmability enables Lattice FPGAs to support the latest models and techniques with astounding efficiency, typically consuming less than 200 mW when running visual AI workloads at 30+ frames per second. In this talk, Hussein Osman, Segment Marketing Director at Lattice Semiconductor, shows how Lattice FPGAs, coupled with the company’s production-proven sensAI solution stack, are being used to quickly develop super-efficient AI implementations that enable groundbreaking features in smart edge devices.
Accelerate All Your Algorithms with the quadric q16 Processor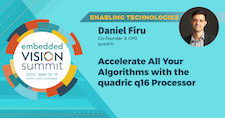
As edge and embedded vision applications increasingly incorporate neural networks, developers are looking to add neural network accelerator functionality to their systems. There is just one problem: we need more than just neural networks in real application pipelines. For most real-world applications, robust algorithm pipelines combine classical image processing and computer vision algorithms for pre-processing (for example, image resizing) and post-processing (such as non-maximal suppression) along with DNNs. These classical algorithms cannot leverage neural network accelerators, and can quickly become performance bottlenecks. In this talk, Daniel Firu, Co-founder and CPO of quadric, introduces the quadric q16 processor, which was designed from the ground up to accelerate a wide variety of image, vision and machine learning algorithms. He highlights the distinctive features of the q16, the architecture behind it, and the associated software development tools. Bringing these all together, he explores use cases illustrating how they solve performance bottlenecks that neural network accelerators do not address.
|
SELF-DRIVING VEHICLE IMPLEMENTATIONS AND FORECASTS |
Autonomous Driving AI Workloads: Technology Trends and Optimization Strategies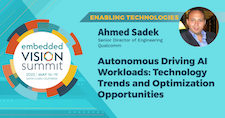
Enabling safe, comfortable and affordable autonomous driving requires solving some of the most demanding and challenging technological problems. From centimeter-level localization to multimodal sensor perception, sensor fusion, behavior prediction, maneuver planning and trajectory planning and control, each one of these functions introduces its own unique challenges that must be solved, verified, tested and deployed on the road. In this talk, Ahmed Sadek, Senior Director of Engineering at Qualcomm, reviews recent trends in AI workloads for autonomous driving as well as promising future directions. He covers AI workloads in camera, radar and lidar perception, AI workloads in environmental modeling, behavior prediction and drive policy. To enable optimized network performance at the edge, quantization and neural architecture optimization are typically performed either during training or post-training. Sadek also covers the importance of hardware-aware quantization and network architecture optimization, and introduces the innovation done by Qualcomm in these areas.
From ADAS to AD, Processor and Car Architecture Evolution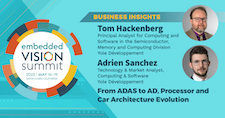
Few markets are as impactful to the future of the semiconductor market as advanced driver assistance systems (ADAS) and the evolution of autonomous driving (AD). At the heart of the race toward AD is the concept of reshaping the car’s embedded systems around the capabilities of new AI processors, versus evolving embedded systems shaped around the evolving hardware, sensors, and customer attitudes toward the current household car. In this talk, Tom Hackenberg, Principal Analyst for Computing and Software in the Semiconductor, Memory and Computing Division, and Adrien Sanchez, Technology and Market Analyst for Computing and Software, both of Yole Développement, present Yole Développement’s market analysis of this shift, covering the competitive landscape within classic processors, the emergence of AI acceleration coprocessors, the evolution of the AI-enabled SoCs and high-performance centralized platforms. They also discuss market drivers such as sensor diversity, memory, power, cost, reliability and the competitive landscape between the household car and mobility-as-a-service (MaaS).
|
UPCOMING INDUSTRY EVENTS |
Putting Activations on a Diet – Or Why Watching Your Weights Is Not Enough – Perceive Webinar: November 10, 2022, 9:00 am PT
How to Successfully Deploy Deep Learning Models on Edge Devices – Deci Webinar: December 13, 2022, 9:00 am PT
Embedded Vision Summit: May 22-25, 2023, Santa Clara, California
More Events
|
FEATURED NEWS |
AMD Unveils Advanced Graphics Cards Built on Groundbreaking RDNA 3 Architecture with Chiplet Design
Quadric’s New Chimera GPNPU Processor IP Blends NPU and DSP into New Category of Hybrid SoC Processor
New Time-of-Flight Camera Model Complements Basler’s 3D Portfolio
Teledyne Expands Its Genie Nano Portfolio with New 10GigE Cameras
SmartCow Releases AI SuperCam and Box PC Powered by NVIDIA Jetson AGX Orin for Next-generation Edge Applications
More News
|
EDGE AI AND VISION PRODUCT OF THE YEAR WINNER SHOWCASE |
Synopsys’ DesignWare ARC EV7xFS Processor IP for Functional Safety (Best Automotive Solution)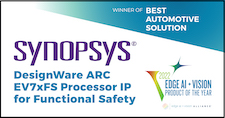
Synopsys’ DesignWare ARC EV7xFS Processor IP for Functional Safety is the 2022 Edge AI and Vision Product of the Year Award winner in the Automotive Solutions category. The EV7xFS is a unique multi-core SIMD vector digital signal processing (DSP) solution that combines seamless scalability of computer vision, DSP and AI processing with state-of-the-art safety features for real-time applications in next-generation automobiles. This processor family scales from a single core EV71FS to a dual core EV72FS and a quad core EV74FS. The multicore vector DSP products include L1 cache coherence and a software tool chain that supports OpenCL C or C/C++ and automatically partitions algorithms across multiple cores. All cores share the same programming model and one set of development tools, ARC MetaWare EV Development Toolkit for Safety. The EV7xFS family includes unique fine-grained power management for maximizing power efficiency. AI is supported in the vector DSP cores and can be scaled to higher levels of performance with optional neural network accelerators. The EV7xFS processors were built with a ground-up approach applying the latest state-of-the-art safety concepts to the design of the EV7xFS architecture. This approach was critical to achieve the ideal balance of performance, power, area and safety for use in today’s automotive SoCs requiring safety levels up to ASIL D for autonomous driving.
Please see here for more information on Synopsys’ DesignWare ARC EV7xFS Processor IP for Functional Safety. The Edge AI and Vision Product of the Year Awards celebrate the innovation of the industry’s leading companies that are developing and enabling the next generation of edge AI and computer vision products. Winning a Product of the Year award recognizes a company’s leadership in edge AI and computer vision as evaluated by independent industry experts. |