LETTER FROM THE EDITOR |
Dear Colleague,
Tomorrow, Thursday, March 7 at 9 am PT, FRAMOS will deliver the free webinar “Build vs Buy: Navigating Optical Image Sensor Module Complexities” in partnership with the Edge AI and Vision Alliance. Among the diversity of development options available in today’s computer vision industry, the decision of whether to build or buy an optical image sensor module is particularly pivotal. This webinar, presented by FRAMOS’ Nathan Dinning, Director of Product Management, and Prashant Mehta, Technical Imaging Expert, will discuss the challenges companies face when opting to develop their own modules, as well as alternative approaches. Dinning and Mehta will explore common development hurdles, including the details of electrical design as characterized using the EMVA1288 standard, and focusing on implementation aspects such as dark signal non-uniformities. Also discussed in detail are other key development topics including:
- The critical alignment of sensor and lens parameters such as chief ray angles (CRA) and the modulation transfer function (MTF),
- The complexities involved in implementing custom image processing pipelines based on often-proprietary and closed technology foundations, and
- The stringent requirements of module production, including statistical quality control across large unit volumes.
Not every company has the resources or expertise to tackle suchintricate development. This is where companies like FRAMOS, which do themodule development work on behalf of their customers, come into play. FRAMOS’ off-the-shelf, ready-to-use FSM:GO modules alleviate the challenges of do-it-yourself modules while also providing the flexibility typically associated only with custom projects. Dinning and Mehta will describe, for example, how these modules offer a range of off-the-shelf lens selections with various focus distances, combining convenience with customization. Attendees will gain insights into how FRAMOS’ solutions can streamline their computer vision system development, as well as resources for further exploration. A question-and-answer session will follow the presentation. For more information and to register, please see the event page.
Brian Dipert
Editor-In-Chief, Edge AI and Vision Alliance |
MODEL OPTIMIZATION TECHNIQUES |
Introduction to Optimizing ML Models for the Edge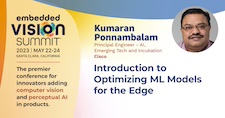
Edge computing opens up a new world of use cases for deep learning across numerous markets, including manufacturing, transportation, healthcare and retail. Edge deployments also pose new challenges for machine learning, not seen in cloud deployments. Constrained resources, tight latency requirements, limited bandwidth and unreliable networks require us to rethink how we build, deploy and operate deep learning models at the edge. In this 2023 Embedded Vision Summit presentation, Kumaran Ponnambalam, Principal Engineer of AI, Emerging Tech and Incubation at Cisco Systems, introduces proven techniques, patterns and best practices for optimizing computer vision models for the edge. He covers quantization, pruning, low-rank approximation and knowledge distillation, explaining how they work and when to use them. And he touches on how your choice of ML framework and processor affect how you use these optimization techniques.
Learning Compact DNN Models for Embedded Vision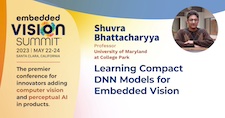
In this 2023 Summit talk, Shuvra Bhattacharyya, Professor at the University of Maryland at College Park, explores methods to transform large deep neural network (DNN) models into effective compact models. The transformation process that he focuses on—from large to compact DNN form—is referred to as pruning. Pruning involves the removal of neurons or parameters from a neural network. When performed strategically, pruning can lead to significant reductions in computational complexity without significant degradation in accuracy. It is sometimes even possible to increase accuracy through pruning. Pruning provides a general approach for facilitating real-time inference in resource-constrained embedded computer vision systems. Bhattacharyya provides an overview of important aspects to consider when applying or developing a DNN pruning method and presents details on a recently introduced pruning method called NeuroGRS. NeuroGRS considers structures and trained weights jointly throughout the pruning process and can result in significantly more compact models compared to other pruning methods.
|
SENSING AND PROCESSING ADVANCEMENTS |
Efficient Neuromorphic Computing with Dynamic Vision Sensor, Spiking Neural Network Accelerator and Hardware-aware Algorithms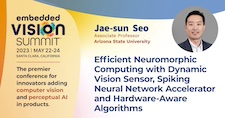
Spiking neural networks (SNNs) mimic biological nervous systems. Using event-driven computation and communication, SNNs achieve very low power consumption. However, two important issues have persisted. First, directly training SNNs has not yielded competitive inference accuracy. Second, non-spike inputs must be converted to spike trains, resulting in long latency. Recently, SNN algorithm accuracy has improved significantly, aided by new training techniques, and commercial event-based dynamic vision sensors (DVSs) have emerged. Integrating a spike-based DVS with an SNN accelerator is a promising approach for end-to-end, event-driven operations. We also need accurate and hardware-aware SNN algorithms that can be directly trained with input spikes from a DVS while reducing storage and compute requirements. In this 2023 Summit talk, Jae-sun Seo, Associate Professor at Arizona State University, introduces the characteristics, opportunities and challenges of SNNs, and presents results from projects utilizing neuromorphic algorithms and custom hardware.
Sensor Fusion Techniques for Accurate Perception of Objects in the Environment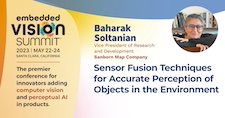
Increasingly, perceptual AI is being used to enable devices and systems to obtain accurate estimates of object locations, speeds and trajectories. In demanding applications, this is often best done using a heterogeneous combination of sensors (e.g., vision, radar, LiDAR). In this 2023 Summit presentation, Baharak Soltanian, Vice President of Research and Development for the Sanborn Map Company, introduces techniques for combining data from multiple sensors to obtain accurate information about objects in the environment. Soltanian briefly introduces the roles played by Kalman filters, particle filters, Bayesian networks and neural networks in this type of fusion. She then examines alternative fusion architectures, such as centralized and decentralized approaches, to better understand the trade-offs associated with different approaches to sensor fusion as used to enhance the ability of machines to understand their environment.
|
UPCOMING INDUSTRY EVENTS |
Build vs Buy: Navigating Optical Image Sensor Module Complexities – FRAMOS Webinar: March 7, 2024, 9:00 am PT
Embedded Vision Summit: May 21-23, 2024, Santa Clara, California
More Events
|
FEATURED NEWS |
Cadence Expands Its Tensilica Vision Family with a Radar Accelerator and New DSPs Optimized for Automotive Applications
e-con Systems Launches a New Rugged PoE HDR Camera with Cloud-based Device Management for Enhanced Outdoor Imaging
Intel Announces a New Edge Platform for Scaling AI Applications
Qualcomm’s AI Hub Brings the Generative AI Revolution to Devices and Empowers Developers
Micron Technology Commences Volume Production of High-bandwidth Memory to Accelerate the Growth of AI
More News
|
EDGE AI AND VISION PRODUCT OF THE YEAR WINNER SHOWCASE |
Deci Deep Learning Development Platform (Best Edge AI Developer Tool)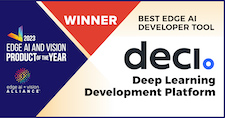
Deci’s Deep Learning Development Platform is the 2023 Edge AI and Vision Product of the Year Award winner in the Edge AI Developer Tools category. Deci’s deep learning development platform empowers AI developers with a new way to develop production grade computer vision models. With Deci, teams simplify and accelerate the development process with advanced tools to build, train, optimize, and deploy highly accurate and efficient models to any environment including edge devices. Models developed with Deci deliver a combination of high accuracy, speed and compute efficiency and therefore allow teams to unlock new applications on resource constrained edge devices and migrate workloads from cloud to edge. Also, Deci enables teams to shorten development time and lower operational costs by up to 80%. Deci’s platform is powered by its proprietary Automated Neural Architecture Construction (AutoNAC) technology, the most advanced algorithmic optimization engine that generates best-in-class deep learning model architectures for any vision-related task. With AutoNAC, teams can easily build custom, hardware-aware production grade models that deliver better than state-of-the-art performance.
Please see here for more information on Deci’s Deep Learning Development Platform. The Edge AI and Vision Product of the Year Awards celebrate the innovation of the industry’s leading companies that are developing and enabling the next generation of edge AI and computer vision products. Winning a Product of the Year award recognizes a company’s leadership in edge AI and computer vision as evaluated by independent industry experts. |