Processors for Embedded Vision
THIS TECHNOLOGY CATEGORY INCLUDES ANY DEVICE THAT EXECUTES VISION ALGORITHMS OR VISION SYSTEM CONTROL SOFTWARE
This technology category includes any device that executes vision algorithms or vision system control software. The following diagram shows a typical computer vision pipeline; processors are often optimized for the compute-intensive portions of the software workload.

The following examples represent distinctly different types of processor architectures for embedded vision, and each has advantages and trade-offs that depend on the workload. For this reason, many devices combine multiple processor types into a heterogeneous computing environment, often integrated into a single semiconductor component. In addition, a processor can be accelerated by dedicated hardware that improves performance on computer vision algorithms.
General-purpose CPUs
While computer vision algorithms can run on most general-purpose CPUs, desktop processors may not meet the design constraints of some systems. However, x86 processors and system boards can leverage the PC infrastructure for low-cost hardware and broadly-supported software development tools. Several Alliance Member companies also offer devices that integrate a RISC CPU core. A general-purpose CPU is best suited for heuristics, complex decision-making, network access, user interface, storage management, and overall control. A general purpose CPU may be paired with a vision-specialized device for better performance on pixel-level processing.
Graphics Processing Units
High-performance GPUs deliver massive amounts of parallel computing potential, and graphics processors can be used to accelerate the portions of the computer vision pipeline that perform parallel processing on pixel data. While General Purpose GPUs (GPGPUs) have primarily been used for high-performance computing (HPC), even mobile graphics processors and integrated graphics cores are gaining GPGPU capability—meeting the power constraints for a wider range of vision applications. In designs that require 3D processing in addition to embedded vision, a GPU will already be part of the system and can be used to assist a general-purpose CPU with many computer vision algorithms. Many examples exist of x86-based embedded systems with discrete GPGPUs.
Digital Signal Processors
DSPs are very efficient for processing streaming data, since the bus and memory architecture are optimized to process high-speed data as it traverses the system. This architecture makes DSPs an excellent solution for processing image pixel data as it streams from a sensor source. Many DSPs for vision have been enhanced with coprocessors that are optimized for processing video inputs and accelerating computer vision algorithms. The specialized nature of DSPs makes these devices inefficient for processing general-purpose software workloads, so DSPs are usually paired with a RISC processor to create a heterogeneous computing environment that offers the best of both worlds.
Field Programmable Gate Arrays (FPGAs)
Instead of incurring the high cost and long lead-times for a custom ASIC to accelerate computer vision systems, designers can implement an FPGA to offer a reprogrammable solution for hardware acceleration. With millions of programmable gates, hundreds of I/O pins, and compute performance in the trillions of multiply-accumulates/sec (tera-MACs), high-end FPGAs offer the potential for highest performance in a vision system. Unlike a CPU, which has to time-slice or multi-thread tasks as they compete for compute resources, an FPGA has the advantage of being able to simultaneously accelerate multiple portions of a computer vision pipeline. Since the parallel nature of FPGAs offers so much advantage for accelerating computer vision, many of the algorithms are available as optimized libraries from semiconductor vendors. These computer vision libraries also include preconfigured interface blocks for connecting to other vision devices, such as IP cameras.
Vision-Specific Processors and Cores
Application-specific standard products (ASSPs) are specialized, highly integrated chips tailored for specific applications or application sets. ASSPs may incorporate a CPU, or use a separate CPU chip. By virtue of their specialization, ASSPs for vision processing typically deliver superior cost- and energy-efficiency compared with other types of processing solutions. Among other techniques, ASSPs deliver this efficiency through the use of specialized coprocessors and accelerators. And, because ASSPs are by definition focused on a specific application, they are usually provided with extensive associated software. This same specialization, however, means that an ASSP designed for vision is typically not suitable for other applications. ASSPs’ unique architectures can also make programming them more difficult than with other kinds of processors; some ASSPs are not user-programmable.
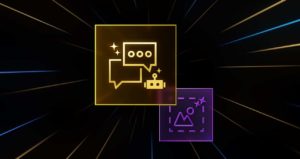
AI Decoded From GTC: The Latest Developer Tools and Apps Accelerating AI on PC and Workstation
This blog post was originally published at NVIDIA’s website. It is reprinted here with the permission of NVIDIA. Next Chat with RTX features showcased, TensorRT-LLM ecosystem grows, AI Workbench general availability, and NVIDIA NIM microservices launched. Editor’s note: This post is part of the AI Decoded series, which demystifies AI by making the technology more
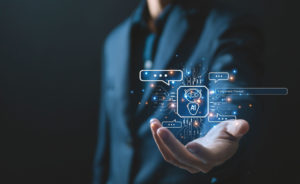
The Rise of Generative AI: A Timeline of Breakthrough Innovations
This blog post was originally published at Qualcomm’s website. It is reprinted here with the permission of Qualcomm. Explore the most pivotal advancements that shaped the landscape of generative AI From Alan Turing’s pioneering work to the cutting-edge transformers of the present, the field of generative artificial intelligence (AI) has witnessed remarkable breakthroughs — and
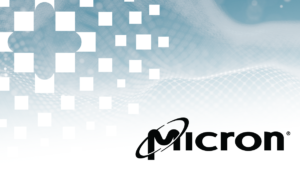
Micron’s Full Suite of Automotive-grade Solutions Qualified for Qualcomm Automotive Platforms to Power AI in Vehicles
Micron’s automotive memory and storage enable central compute, digital cockpit and advanced driver-assistance systems for Qualcomm customers NUREMBERG, Germany, April 10, 2024 (GLOBE NEWSWIRE) — Embedded World — Micron Technology, Inc. (Nasdaq: MU), today announced that it has qualified a full suite of its automotive-grade memory and storage solutions for Qualcomm Technologies Inc.’s Snapdragon® Digital Chassis™,
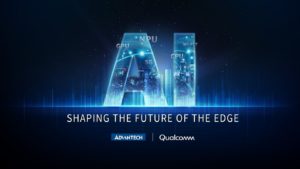
Advantech Establishes Collaboration with Qualcomm to Shape the Future of the Edge
Taipei, Taiwan and Nuremberg, Germany, 10th April 2024 — Today, at Embedded World, Advantech proudly announced its strategic collaboration with Qualcomm Technologies, Inc. to revolutionize the edge computing landscape. This effort, combining AI expertise, high-performance computing, and industry-leading connectivity, is set to propel innovation for industrial computing. This collaboration establishes an open and diverse edge
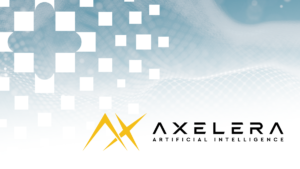
Axelera Uses oneAPI Construction Kit to Rapidly Enable Open Standards Programming for the Metis AIPU
AI applications have an endless hunger for computational power. Currently, increasing the sizes of the models and cranking up the number of parameters has not quite yet reached the point of diminishing returns and thus the ever growing models still yield better performance than their predecessors. At the same time, new areas for application of
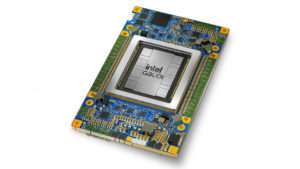
Intel Breaks Down Proprietary Walls to Bring Choice to Enterprise GenAI Market
Intel Gaudi 3 AI accelerator brings global enterprises choice for generative AI, building on the performance and scalability of its Gaudi 2 predecessor. What’s New: At Intel Vision, Intel introduces the Intel® Gaudi® 3 AI accelerator, which delivers 4x AI compute for BF16, 1.5x increase in memory bandwidth, and 2x networking bandwidth for massive system
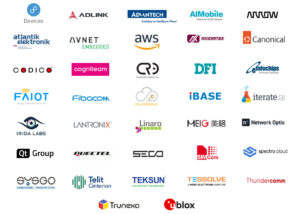
Qualcomm Announces Breakthrough Wi-Fi Technology and Introduces New AI-ready IoT and Industrial Platforms at Embedded World 2024
Highlights: Qualcomm Technologies’ leadership in connectivity, high performance, low power processing, and on-device AI position it at the center of the digital transformation of many industries. Introducing new industrial and embedded AI platforms, as well as micro-power Wi-Fi SoC—helping to enable intelligent computing everywhere. The Company is looking to expand its portfolio to address the
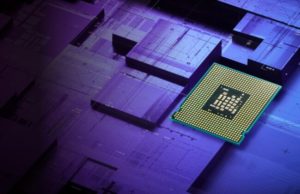
Imagination’s New Catapult CPU is Driving RISC-V Device Adoption
Imagination APXM-6200 CPU: The performance-dense RISC-V application processor for Intelligent, Consumer and Industrial Applications 08 April 2024 – Imagination Technologies today unveils the next product in the Catapult CPU IP range, the Imagination APXM-6200 CPU: a RISC-V application processor with compelling performance density, seamless security and the artificial intelligence capabilities needed to support the compute
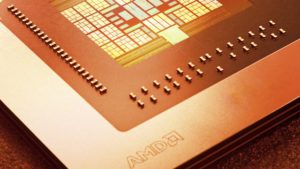
AMD Extends Leadership Adaptive SoC Portfolio with New Versal Series Gen 2 Devices Delivering End-to-end Acceleration for AI-driven Embedded Systems
First devices in AMD Versal Series Gen 2 portfolio target up to 3x higher TOPs-per-watt with next-gen AI Engines and up to 10x more CPU-based scalar compute than first generation Subaru among first customers to announce plans to deploy AMD Versal AI Edge Series Gen 2 to power next-gen ‘EyeSight’ ADAS vision system NUREMBERG, Germany,
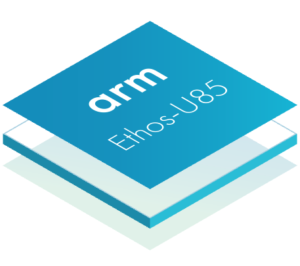
Arm Accelerates Edge AI with Latest Generation Ethos-U NPU and New IoT Reference Design Platform
News highlights: New Arm Ethos-U85 NPU delivers 4x performance uplift for high performance edge AI applications such as factory automation and commercial or smart home cameras New Arm IoT Reference Design Platform, Corstone-320, brings together leading-edge embedded IP with virtual hardware to accelerate deployment of voice, audio, and vision systems With a global ecosystem of
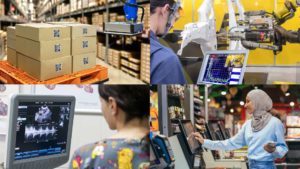
Intel and Altera Announce Edge and FPGA Offerings for AI at Embedded World
New edge-optimized processors and FPGAs bring AI everywhere across edge computing markets including retail, industrial and healthcare. What’s New: Today at Embedded World, Intel and Altera, an Intel Company, announced new edge-optimized processors, FPGAs and programmable market-ready solutions extending powerful AI capabilities into edge computing. These products will power AI-enabled edge devices applicable to industries across
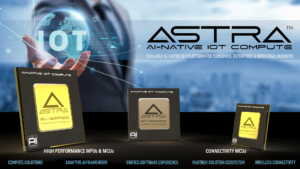
Synaptics Astra AI-native IoT Platform Launches with SL-series Embedded Processors and Machina Foundation Series Development Kit
Highly integrated Linux® and Android™ SoCs and dev kit are optimized for consumer, enterprise, and industrial applications and deliver an ‘out-of-the-box’ edge AI experience. San Jose, CA, April 8, 2024 – Synaptics® Incorporated (Nasdaq: SYNA) today launched the Synaptics Astra platform with the SL-Series of embedded AI-native Internet of Things (IoT) processors and the Astra
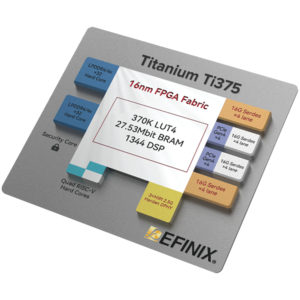
Efinix’s Titanium Ti375 Now Sampling: Unlocking and Delivering Mainstream Edge Intelligence Innovation
Titanium Ti375 FPGAs set the bar for high performance compute at the edge for smart devices with low power, paving the way for edge intelligence April 08, 2024 12:01 AM Eastern Daylight Time–CUPERTINO, Calif.–(BUSINESS WIRE)–Efinix®, an innovator in programmable logic solutions, today announced its Titanium Ti375 high performance FPGA is now shipping in sample quantities
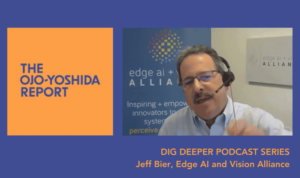
What Changed From VCRs to Software-defined Vision?
The Edge AI and Vision Alliance’s Jeff Bier discusses how advances in embedded systems’ basic building blocks are fundamentally changing their nature. The Ojo-Yoshida Report is launching the second in our Dig Deeper video podcast series, this issue focusing on “Embedded Basics.” Our guest for the inaugural episode is Jeff Bier, founder, Edge AI and Embedded Vision
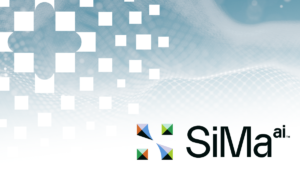
SiMa.ai Secures Funds and Readies New Generative Edge AI Platform
Oversubscribed $70M round led by Maverick Capital increases SiMa.ai’s total amount raised to $270M; funding will speed release of next generation AI/ML chip to power multimodal generative AI at the edge SAN JOSE, Calif., April 4, 2024 – SiMa.ai, the software-centric, embedded edge machine learning system-on-chip company, today announced it has raised an additional $70M
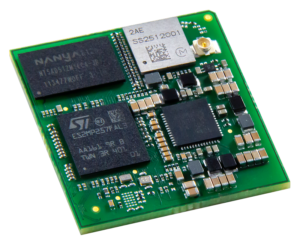
Digi International to Unveil the New Digi ConnectCore MP25 System-on-module for Next-gen Computer Vision Applications at Embedded World 2024
Versatile, wireless and secure system-on-module based on the STMicroelectronics STM32MP25 processor improves efficiency, reduces costs and enables edge processing for innovative new devices MINNEAPOLIS, April 4, 2024 – Digi International (NASDAQ: DGII, www.digi.com), a leading global provider of Internet of Things (IoT) connectivity products and services, is poised to introduce the wireless and highly power-efficient
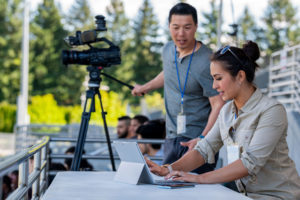
How to Enable Efficient Generative AI for Images and Videos
This blog post was originally published at Qualcomm’s website. It is reprinted here with the permission of Qualcomm. Find out how Qualcomm AI Research reduced the latency of generative AI Generative artificial intelligence (AI) is rapidly changing the way we create images, videos, and even three-dimensional (3D) content. Leveraging the power of machine learning, generative
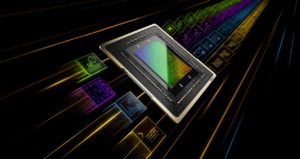
AI Decoded: Demystifying AI and the Hardware, Software and Tools That Power It
This blog post was originally published at NVIDIA’s website. It is reprinted here with the permission of NVIDIA. RTX AI PCs and workstations deliver exclusive AI capabilities and peak performance for gamers, creators, developers and everyday PC users. With the 2018 launch of RTX technologies and the first consumer GPU built for AI — GeForce
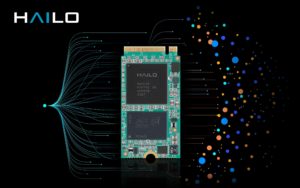
Hailo Closes New $120 Million Funding Round and Debuts Hailo-10, A New Powerful AI Accelerator Bringing Generative AI to Edge Devices
Hailo’s funding now exceeds $340 million as the company introduces its newest AI accelerator specifically designed to process LLMs at low power consumption for the personal computer and automotive industries, bringing generative AI to the edge. TEL AVIV, Israel, April 2, 2024 — Hailo, the pioneering chipmaker of edge artificial intelligence (AI) processors, today announced
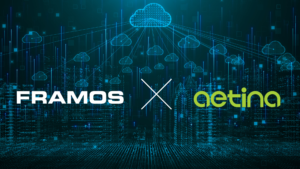
Adding Vision to Your Embedded Devices? Simplify Your Imaging Journey with FRAMOS and Aetina
2nd of April, 2024 – As Elite members of the NVIDIA Partner Network and part of the NVIDIA Jetson ecosystem, FRAMOS and Aetina have combined their strengths to showcase solutions for embedded device innovators at ISC West 2024, April 9-12 at The Venetian Expo, Las Vegas. This cooperation brings the collective benefits of FRAMOS’ FSM:GO